Molecular Docking, ADME and SAR Analysis of 383 Phytochemicals in the Quest for Lead Antidiabetic Inhibitors Targeting α-Amylase and α-Glucosidase Enzymes
DOI:
https://doi.org/10.26538/tjdr/v2i1.2Keywords:
Αlpha-amylase, Αlpha-glucosidase, Diabetes, Structure-activity relationship, Flavonoids, Amentoflavone.Abstract
Purpose: Diabetes mellitus remains a global health challenge, necessitating the exploration of safe and effective therapeutic agents. The inhibition of α-amylase and α-glucosidase enzymes plays a crucial role in controlling postprandial hyperglycemia. While synthetic inhibitors exist, they often cause gastrointestinal side effects, prompting the search for novel, naturally derived inhibitors. This study evaluates the molecular interactions of 383 phytochemicals, focusing on alkaloids, terpenes, and flavonoids, for their potential as lead antidiabetic compounds.
Methods: Molecular docking studies were performed using AutoDock Vina to assess the binding affinity of selected phytochemicals against human pancreatic α-amylase (PDB ID: 5EMY) and α-glucosidase (PDB ID: 2QMJ). The physicochemical and pharmacokinetic properties of the top-performing compounds were analyzed using SwissADME, following Lipinski’s Rule of Five and Verber’s rules. Structure-activity relationship (SAR) analysis was conducted to elucidate key functional groups responsible for enzyme inhibition.
Results: Flavonoids exhibited superior inhibitory potential, with binding affinities outperforming the standard inhibitor Acarbose. The most potent compounds, Amentoflavone (-9.5 kcal/mol), Hesperidin (-9.5 kcal/mol), Eriocitrin (-9.5 kcal/mol), and Diosmin (-9.4 kcal/mol), showed strong interactions with key amino acid residues. SAR analysis highlighted the significance of glycosylation and flavone / flavanol moieties in enhancing binding affinity. ADME analysis revealed favorable pharmacokinetic properties, with Amentoflavone demonstrating the highest synthetic accessibility and drug-likeness.
Conclusion: This study identifies flavonoids as promising dual inhibitors of α-amylase and α-glucosidase, with Amentoflavone emerging as a lead candidate for further development as a novel antidiabetic agent, contributing to the search for safer alternatives to conventional therapies.
Downloads
References
1. Sugandh FN, Chandio M, Raveena FN, Kumar L, Karishma FN, Khuwaja S, Memon UA, Bai K, Kashif M, Varrassi G, Khatri M, Kumar S. Advances in the Management of Diabetes Mellitus: A Focus on Personalized Medicine. Cureus. 2023; 15(8): e43697. https://doi.org/10.7759/cureus.43697
2. Dirir AM, Daou M, Yousef AF, & Yousef LF. A review of alpha-glucosidase inhibitors from plants as potential candidates for the treatment of type-2 diabetes. Phytochem Rev. 2022; 21(4): 1049–1079.
3. Yao Y, Liu J, Miao Q, Zhu X, Sun L, Hua W, Zhang N, Huang G, Ruan R, Cheng Y, Mi S. Inhibition and effect of almond hull extract on activities of α-amylase and α-glucosidase, and postprandial glucose in normal SD rats. J Funct Foods. 2024; 123 106624. https://doi.org/10.1016/j.jff.2024.106624
4. Shehadeh MB, Suaifan GA, Abu-odeh AM. Plants' secondary metabolites as blood glucose-lowering molecules. Molecules. 2021; 26(14): 4333. https://doi.org/10.3390/molecules26144333
5. Yang L & Stöckigt J. Trends for diverse production strategies of plant medicinal alkaloids. Nat Prod Rep. 2010; 10 (27): 1469-1479. http://dx.doi.org/10.1039/C005378C
6. Iqbal A, Farrukh A, Mohammad O. Modern Phytomedicine, Turning Medicinal Plants into Drugs. 12-13, WILEY-VCH Verlag GmbH & Co. KGaA, Weinheim, 2006.
7. Shamsudin NF, Ahmed QU, Mahmood S, Shah SA, Sarian MN, Khattak MM, Khatib A, Sabere AS, Yusoff YM, Latip J. Flavonoids as Antidiabetic and Anti-Inflammatory Agents: A Review on Structural Activity Relationship-Based Studies and Meta-Analysis. Int J Mol Sci. 2022; 23(20): 12605. https://doi.org/10.3390/ijms232012605
8. Ullah A, Munir S, Badshah SL, Khan N, Ghani L, Poulson BG, Emwas A, Jaremko M. Important flavonoids and their role as a therapeutic agent. Molecules. 2020; 25(22): 5243. https://doi.org/10.3390/molecules25225243
9. Lipinski CA. Lead- and drug-like compounds: the rule-of-five revolution. Drug Discov Today Technol. 2004; 1(4): 337–341. https://doi.org/10.1016/j.ddtec.2004.11.007
10. Veber DF, Johnson SR, Cheng HY, Smith BR, Ward KW, Kopple KD, Molecular properties that influence the oral bioavailability of drug candidates. J Med Chem. 2002; 45 (12): 2615-2623
11. Cochran WG. 1977. Sampling Techniques. 3rd ed. New York: John Wiley & Sons.
12. Zhang S, Wang Y, Han L, Fu X, Wang S, Li W, Han W. Targeting N-Terminal human Maltase-Glucoamylase to unravel possible inhibitors using molecular docking, molecular dynamics simulations, and adaptive steered molecular dynamics simulations. Front Chem. 2021; 9. 711242. https://doi.org/10.3389/fchem.2021.711242
13. Burley SK, Berman HM, Bhikadiya C, Bi C, Chen L, Di Costanzo L, Christie C, Dalenberg K, Duarte JM, Dutta S, Feng Z, Ghosh S, Goodsell DS, Green RK, Guranović V, Guzenko D, Hudson BP, Kalro T, Liang Y, Zardecki C. RCSB Protein Data Bank: biological macromolecular structures enabling research and education in fundamental biology, biomedicine, biotechnology and energy. NAR. 2018; 47(D1): D464–D474. https://doi.org/10.1093/nar/gky1004
14. Kim S, Thiessen PA, Bolton EE, Chen J, Fu G, Gindulyte A, Bryant SH. PubChem Substance and Compound databases. NAR. 2015; 44(D1): D1202–D1213. https://doi.org/10.1093/nar/gkv951
15. O’Boyle, NM, Banck M, James CA, Morley C, Vandermeersch T, Hutchison GR. Open Babel: An open chemical toolbox. J Cheminform. 2011; 3(1): 33. https://doi.org/10.1186/1758-2946-3-33
16. Trott O, Olson AJ. AutoDock Vina: Improving the speed and accuracy of docking with a new scoring function, efficient optimization, and
multithreading. J. Comput. Chem. 2010; 31(2): 455-461. https://doi.org/10.1002/jcc.21334
17. Schrödinger L, Delano W. PyMOL. The PyMOL Molecular Graphics System, Version 2. Schrödinger, LLC: New York, NY, USA. (2020).
18. Biovia, D.S., & Systèmes, D. Discovery Studio Modeling Environment (Version 53). Dassault Systèmes. (2016).
19. SwissADME: A free web tool to evaluate pharmacokinetics, drug-likeness and medicinal chemistry friendliness of small molecules. Sci. Rep. 2017; 7:42717.
20. Ogunwa, T. Insights into interaction profile and inhibitory potential of amentoflavone with α-glucosidase, tyrosinase and 15-lipoxygenase as validated therapeutic targets. J Syst Biol Proteome Res. 2018; 2(1). 10-20.
21. Swargiary A, Roy MK, Mahmud S. Phenolic compounds as α-glucosidase inhibitors: a docking and molecular dynamics simulation study. J Biomol Struct Dyn. 2023; 41(9): 3862-3871. doi: 10.1080/07391102.2022.2058092.
22 .Ortega JT, Suárez AI, Serrano ML, Baptista J, Pujol FH, Rangel HR. The role of the glycosyl moiety of myricetin derivatives in anti-HIV-1 activity in vitro. AIDS Res Ther. 2017; 14, 57. https://doi.org/10.1186/s12981-017-0183-6
23. Daina A, Michielin O, Zoete V. iLOGP: A simple, robust, and efficient description of n-octanol/water partition coefficient for drug design using the GB/SA approach. J Chem Inf Model. 2014; 54(12): 3284–3301. https://doi.org/10.1021/ci500467k
24. Durán-iturbide NA, Díaz-eufracio BI, Medina-Franco JL. In Silico ADME/Tox Profiling of Natural Products: A Focus on BIOFACQUIM Medina-Franco. ACS, Omega. 2020; 5 (26): 16076-16084 DOI: 10.1021/acsomega.0c01581
25. Lewandowski W, Lewandowska H, Golonko A, Swiderski G, Swislocka R, Kalinowska M. Correlations between molecular structure and biological activity in "logical series" of dietary chromone derivatives. PLoS ONE. 2020; 15(8): e0229477. https://link.gale.com/apps/doc/A633157774/HRCA?u=anon~d7180f70&sid=googleScholar&xid=0e4e5bf8
26. Zhuang W, Wang Y, Cui P, Xing L, Lee J, Kim D, Jiang H, Oh Y. Applications of π-π stacking interactions in the design of drug-delivery systems. JCR. 2018; 294: 311–326. https://doi.org/10.1016/j.jconrel.2018.12.014
27. Odhar HA, Hashim FA, Humad SS. Molecular docking analysis and dynamics simulation of salbutamol with the monoamine oxidase B (MAO-B) enzyme. Bioinformation. 2022; 18(3): 304-309. https://doi.org/10.6026/97320630018304
28. Gómez-jeria JS, Robles-navarro A, Kpotin G, Garrido-sáez N, Nelson GD. Some remarks about the relationships between the common skeleton concept within the Klopman-Peradejordi-Gómez QSAR method and the weak molecule-site interactions. J Chem Res. 2020; 5(2): 32-52
29. Liang Z, Li QX. π-Cation Interactions in Molecular Recognition: Perspectives on Pharmaceuticals and Pesticides. J Agric Food Chem. 2018; 66(13): 3315–3323. https://doi.org/10.1021/acs.jafc.8b00758
30. Alanzi AR, Alhaidhal BA, Alsulais FM. In-silico exploration of potential PRKG1 Inhibitors: A comprehensive study using MTIOPEN Screening, molecular Docking, and MD simulation. J King Saud Univ Sci. 2024; 36(9): 103372. https://doi.org/10.1016/j.jksus.2024.103372
31. Bitencourt-ferreira G, Veit-acosta M, De azevedo WF. Van der Waals Potential in Protein Complexes. Methods in Mol Biol 2019: 79–91. https://doi.org/10.1007/978-1-4939-9752-7_6
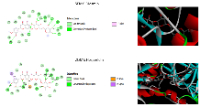
Published
Issue
Section
License

This work is licensed under a Creative Commons Attribution-NonCommercial-ShareAlike 4.0 International License.